
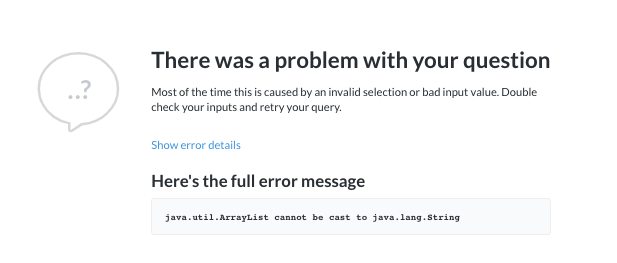
We want good monitoring of the present, which requires appropriately reflecting on past events before tackling work on complex models for predicting the future. To start with, we need to understand the past. With ideally two or three data engineers for each data scientist or data analyst, we want to consolidate tools and optimize for our internal analytics. That's why we decided to start building a Data Analytics Team, focusing our efforts on building a robust plan to solve the data movement problem, which is at the base of the Data Science Hierarchy of Needs. And with it, also the need for analytics is growing. How It’s Going: How Our Data Architecture Grewįast forward to today, we have around 100 people. Developer Experience: GitHub for engineering workflows and maintaining the core of the Airbyte repo.Intercom building customer relationships. Salesforce for handling customer relationship management. Segment for all custom user interactions. User Engagement and Feedback: Orb for credit management.

BigQuery for storing data in a central data warehouse. Metabase and Superset/Preset for dashboards and internal analytics.
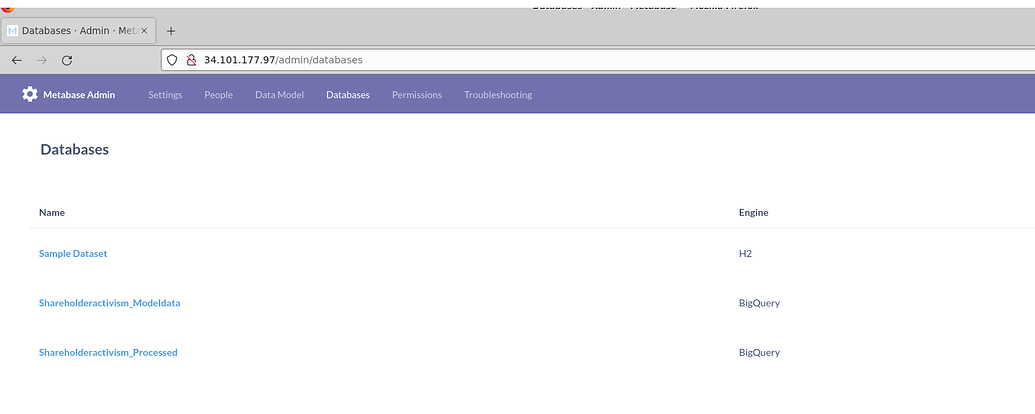
Not all tools are related to the (modern) data stack but helping us collect and assess OKRs and monitoring. If you don’t, it's natural that business users are more likely to choose closed-source as they are less engineering savvy as data engineers build these systems. Especially without a dedicated data team, it's a wise decision to use SaaS tools. In some cases, the tools are not bringing the value you were hoping for thus, you can stop the subscription and not lose lots of expensive time to build it up and manage it. As many of these are closed-source tools, we have to use them if we want to make progress fast. The power of SaaS tools is to quickly build up knowledge in each technology, perfect for validating your assumptions. Overview of initial Data Flow with diverse SaaS tools Below is the data flow diagram that shows different SaaS tools stitched together. Let’s look at what happened at Airbyte during the first year and how our data stack grew. Many other companies might experience similar data flows, especially in a fast-growing company.
#Metabase bigquery full
How Our Data Architecture Grew: Lots of SaaS ToolsĪlthough sharing this early-stage architecture might be intimidating, we want full transparency on how it grew. OKRs are great for building a bridge they give you non-productive pressure to see how your company is heading-aligning everyone on company-wide goals. In terms of the data stack, it meant using simple tools because not everyone is a data engineer.
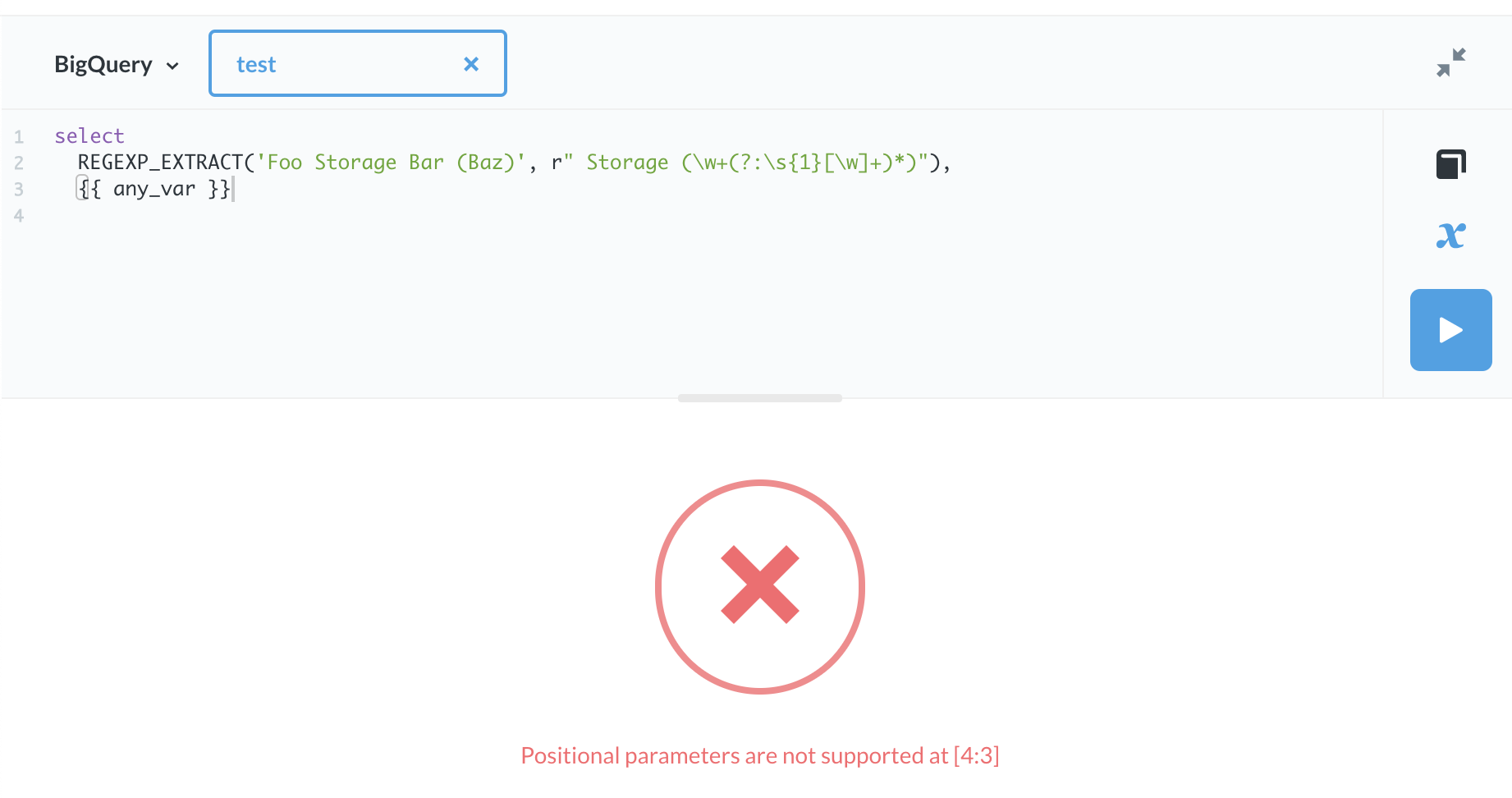
But these are usually the start of analytics and dashboards. These are not only essential for any company to align on commonly defined goals. Airbyte has company-wide OKRs (Objectives and Key Results) to follow from day one, such as daily_active_users, installations_per_day, etc. But before we can measure them, we must define what we want to measure. How it started: Goals, OKRs, KPIsĪs a fast-growing data startup, we are immensely data-driven. We believe these are universal building blocks, and with a commitment to open-source and transparency, we hope it will help you. Uses cases such as adding an orchestrator on top of Airbyte, dbt, Metabase, or how adding a metric layer to our data stack. We start with how the Airbyte data stack started and how it’s going and will publish follow-up articles with hands-on examples of how we solved the challenges mentioned in this article with open-source tooling. We want to take a step back and let you join our data journey, tell you the pain we had and how we are trying to overcome this. You start adding tool after tool you generate copies of data sets in different places-eventually, you lose track of where the data flows through.
